Image recognition SoC designed for automotive applications
Toshiba has announced the development of an image recognition SoC (System on Chip) for automotive applications that implements deep learning accelerator at ten times the speed and four times the power efficiency of Toshiba’s previous product. Details of the technology were reported at the 2019 IEEE International Solid-State Circuits Conference (ISSCC) in San Francisco on 19th February.
Advanced driver assistance systems, such as autonomous emergency braking, offer increasingly advanced capabilities, and implementing them requires image recognition SoC that can recognise road traffic signs and road situations at high speed with low power consumption.
Deep Neural Networks (DNN), algorithms modeled after the neural networks of the brain, perform recognition processing much more accurately than conventional pattern recognition and machine learning, and is widely expected to find utilisation in automotive applications.
However, DNN-based image recognition with conventional processors takes time, as it relies on a huge number of multiply-accumulate (MAC) calculations. DNN with conventional high speed processors also consumes too much power.
Toshiba has overcome this with a DNN accelerator that implement deep learning in hardware. It has three features.
- Parallel MAC units. DNN processing requires many MAC computations. Toshiba’s new device has four processers, each with 256 MAC units. This boosts DNN processing speed.
- Reduced DRAM access. Conventional SoC have no local memory to keep temporal data close to the DNN execution unit and consume a lot of power accessing local memory. Power is also consumed loading the weight data, used for the MAC calculations. In Toshiba’s new device, SRAM are implemented close to the DNN execution unit, and DNN processing is divided into sub-processing blocks to keep temporal data in the SRAM, reducing DRAM access. Additionally, Toshiba has added a decompression unit to the accelerator. Weight data, compressed and stored in DRAM in advance, are loaded through the decompression unit. This reduces the power consumption involved in loading weight data from DRAM.
- Reduced SRAM access. Conventional deep learning needs to access SRAM after processing each layer of DNN, which consumes too much power. The accelerator has a pipelined layer structure in the DNN execution unit of DNN, allowing a series of DNN calculations to be executed by one SRAM access.
The new SoC complies with ISO26262, the global standard for functional safety for automotive applications.
Toshiba will continue to enhance the power efficiency and processing speed of the developed SoC and will start sample shipments of Visconti5, the next generation of Toshiba’s image-recognition processor, in September this year.
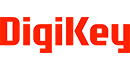