Employing explainable AI in the COVID-19 fight
Examples of artificial intelligence (AI) are all around us. We probably use AI more than we think and, in many ways, take it for granted. Our smartphone assistant is an excellent example of AI. Likewise, face recognition has become a standard unlock feature on new smartphones. However, the technology still requires refinement in critical applications where machine learning (ML) errors could prove costly. Here, Electronic Specifier speak to Gowri Chindalore Head of Technology and Business Strategy, Microcontrollers at NXP, who explains how the company are using explainable AI (xAI), in the fight against COVID-19.
AI, for which ML is a subset, works by training a computer-based neural network model to recognise a given pattern or sound. Once the neural network has completed training, it can then infer a result. For example, if we train a neural network with hundreds of images of dogs and cats, it should then be able to correctly identify a picture as either being a dog or a cat. The network model determines an answer and indicates the class probability of its prediction.
Shortcomings
However, as machine learning-based applications become more deeply ingrained in our daily lives, system developers have become more aware that the current method in which neural networks operate is not necessarily the right approach. Using the above example, if we showed the neural network a picture of a horse, the neural network, trained only to infer either a cat or a dog, would have to decide which one it is going to pick within the class for which it was trained. Of more concern, the likelihood is that it would give an incorrect prediction with a high degree of probability - something you may not even notice. The model has failed silently.
Chindalore commented: “Neural networks are a valiant attempt at mimicking how our brains work - it's an evolving field. We are far away from mimicking the true brain, but the reasoning part of it (the explainability) is part of its evolution towards what a human brain does. It just needs more vectors and capabilities to be added - the least of which is the energy consumption itself. Nature has perfected our brains to be extremely efficient. And today the energy efficiency of neural networks is nowhere close to that of our brains.”
As humans, our approach to a similar scenario would be very different. We use a more reasoned decision-making approach. We would expect the neural network to answer that it did not know or had not seen an image of a horse before. The example above, while very simple, serves to illustrate a flaw in how a neural network must operate in the human world of surprises and uncertainties.
At the level where we are today, this explainability is important, because when a prediction is incorrect, there is a need to be able to go back and establish whether the neural network can give an indication of what went wrong.
If neural network are able to achieve that self-introspection, then that represents a huge step forward. The name given to this ability is uncertainty classification, defined as ‘aleatory’ and ‘epistemic’ uncertainties. In neural network models aleatory is essentially randomness in the input data and epistemic is more systematic errors like the training data is not good enough.
NXP are delivering advanced machine learning solutions for its customers and have been working on an approach termed ‘explainable AI’ (xAI). xAI expands on the inference and probability capabilities of machine learning by adding a more reasoned human-like decision-making approach and the additional dimension of certainty. xAI combines all the benefits of AI with an inference mechanism that is closer to how a human would respond in a situation.
The COVID-19 fight
“As a thought leader in machine learning and given our contribution to the IoT and automotive industry, NXP are always looking at ways to improve capabilities that we provide to our customers,” Chindalore added.
With the unprecedented global COVID-19 pandemic, NXP’s xAI research teams believe that NXP xAI might help enable the rapid detection of the disease in patients. The use of CT radiology and x-ray imaging provides a fast alternative detection capability alongside the prescribed PCR testing and diagnosis protocols. CT and x-ray images could be processed by a suitably trained xAI model to differentiate between clean and infected cases. xAI allows for real-time inference confidence and explainable insights to aid clinical staff in determining the next stage of treatment.
Chindalore continued: “We started seeing that more and more healthcare professionals are beginning to use chest x-rays as one of the ways of recognising COVID-19. PCR tests are by far the most common, however, chest x-rays are also becoming quite popular because the PCR test requires test kits, it takes around a day to turnaround and in many countries around the world, the availability of kits, and the time it takes to send them out to an expert, and get the reading back from the lab etc, was taking more than 24 to 48 hours.”
Chindalore explained that a chest x-ray on the other hand is very quick. And as such, the use of ML to perform chest x-ray readings is on the rise. The concern is the one dimensional nature of current ML neural networks, the false positives or false negatives with high confidence etc, and the lack of explainability. He added: “We recognised that there was opportunity for us to contribute to society by making these explainability algorithms available for chest x-ray recognition.”
This in turn would provide doctors with the confidence in ML-read images, and a high level of variation that can be put in place in order to gain confidence in COVID-19 recognition. Chindalore added that what is needed now is not merely a model, but an extensive data set of chest x-rays that is annotated by experts.
“Our CEO has asked the company to make these models available for any researchers, whether it's a university or hospital, and enable them to work with us to use those models and tweak them as necessary. We are making it available open source. If they want us to work with them on the data set that they have, we will be very happy to work with them. However, if they have privacy concerns, etc. and they would rather take that capability inside their own establishments, train it themselves, and then just consult us on a technical basis, we'll be happy to do that also.”
NXP’s aim is to work with collaborators to make this available and valuable for society during the pandemic and beyond. “We’re calling for collaboration, we are here, can contribute, and can provide technical assistance. We started talking with quite a few universities in the US and Europe. There is quite a bit of an interest, and there is some early collaboration. But nonetheless, we are open to working with more and more people.”
Chindalore was also keen to point out that this development goes far beyond purely the fight against COVID-19. And if we continue developing and making these kinds of capabilities available for society, then future healthcare issues could be handled better and we could have a better response time.
“For example, in countries like Mexico, PCR tests are still very difficult to get hold of,” he continued. “So, in those cases while chest x-rays are more expensive, they are a little faster and instruments are more readily available, so there's still opportunities in this current wave.
“Secondly, if there is a future wave, and if we can perfect xAI, then detection techniques will be much faster. The third aspect is preparing society for advanced detection capabilities for the future. This is a technique that is not solely applicable for COVID-19 alone, you can apply this to improve the predictability and uncertainty of breast cancer detections for example.
“Our effort is going into making the medical community and university researchers more aware of this capability so xAI can be put into more applications than just the correct pandemic.”
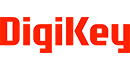